Contact

+49 9131 85 27882

+49 9131 85 27270
Address
Universität Erlangen-Nürnberg
Chair of Computer Science 5 (Pattern Recognition)
Martensstr. 3 91058 Erlangen Germany
Powered by
|
Limited Data Hyperspectral (HS) Remote Sensing (RS) Image Classification
-
The amount of training data that is required to train a classifier scales with the dimensionality of the feature data. In hyperspectral remote sensing, feature data can potentially become very high dimensional. However, the amount of training data is oftentimes limited. Thus, one of the core challenges in hyperspectral remote sensing is how to perform multi-class classification using only relatively few training data points. In this project, we try to address this issue by more informative features and enriching the feature matrix with synthetically generated sample points.
GMM supervectors for limited data HS image classification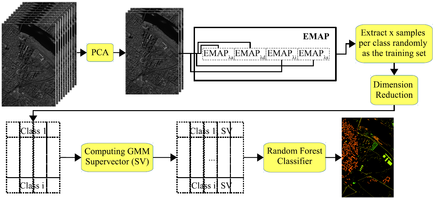 |
Synthetic sample generation for limited data HS image classification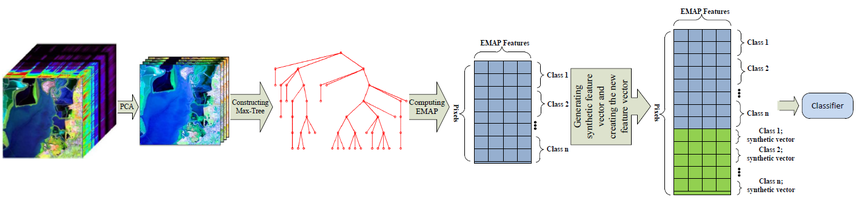 |
International Geoscience and Remote Sensing Symposium (IGARSS), Valencia, Spain, 22-27.07, pp. tbd, 2018 (BiBTeX, Who cited this?)
Computer Analysis of Images and Patterns (17th international Conference on Computer Analysis of Images and Patterns (CAIP)), Ystad, Sweden, 22-24.8.2017, vol. 2, pp. 296-306, 2017 (BiBTeX, Who cited this?)
Signal Processing and Communications Applications Conference (SIU), 2015 23th (23th Signal Processing and Communications Applications Conference (SIU)), Malatya, Turkey, 2015, pp. 653-656, 2015 (BiBTeX, Who cited this?)
Sketch Layer Decomposition in Old Master Drawings Using Hyperspectral Image Analysis
-
Layers of a phantom drawing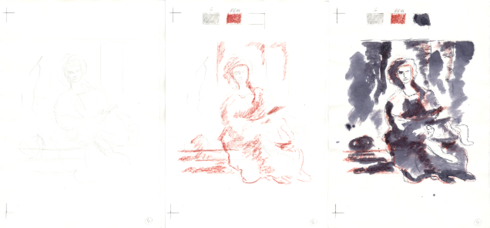 |
Old master drawings were mostly created step by step in several layers using different materials. To art historians and restorers, examination of these layers brings various insights into the artistic work process and helps to answer questions about the object, its attribution and its authenticity.
However, these layers typically overlap and are oftentimes difficult to differentiate with the unaided eye. For example, a common layer combination is red chalk under ink. In this project, we investigate image processing pipeline that operate on hyperspectral images to separate such layers.
International Conference on Image Processing (ICIP), Athens, Greece, 7-10.10, pp. tbd, 2018 (BiBTeX, Who cited this?)
Extended Papers of the International Symposium on Digital Humanities (DH 2016) (Digital Humanities 2016 (DH 2016)), Linnaeus University, Växjö, Sweden, 7-8/2016, vol. 1, pp. 147-160, 2017 (BiBTeX, Who cited this?)
|